・We propose CCPLS, which is a statistical framework for identifying cell–cell communications as the effects of multiple neighboring cell types on cell-to-cell expression variability of highly variable genes (HVGs), based on the spatial transcriptome data.
・For each cell type, CCPLS performs PLS regression modeling and reports coefficients as the quantitative index of the cell–cell communications. Evaluation using simulated data showed our method accurately estimated the effects of multiple neighboring cell types on HVGs.
・Furthermore, applications to the two real datasets demonstrate that CCPLS can extract biologically interpretable insights from the inferred cell–cell communications.
Abstract
The regulation of cell-to-cell expression variability of HVGs via cell–cell communications is still largely unexplored. The recent advent of spatial transcriptome methods has linked gene expression profiles to the spatial context of single cells, which has provided opportunities to reveal the regulation of cell-to-cell expression variability of HVGs via cell–cell communications. We propose CCPLS (Cell-Cell communication analysis by Partial Least square regression modeling), which is a statistical framework for identifying the MIMO (multiple-input and multiple-output) system of cell–cell communications, i.e. gene expression regulation by multiple neighboring cell types, based on the spatial transcriptome data at a single-cell resolution. CCPLS estimates regulation on cell-to-cell expression variation of HVGs via cell–cell communications not limited to ligand–receptor pairs. The core of CCPLS is PLS regression modeling performed by setting the HVGs expression values as the responsive variables and the neighboring cell-type scores as the explanatory variables for each cell type. After the statistical evaluation, CCPLS performs HVG clustering, which is reported with coefficients as the direction and degree of regulation in the cell–cell communication. Evaluation using simulated data showed accurate estimation by CCPLS. The application of CCPLS to the two real spatial transcriptome datasets demonstrated the capability to extract biologically interpretable insights.
Benefit
In this study, we developed an information analysis method called CCPLS to estimate the influence of neighboring cells on each other’s gene expression, using spatial transcriptome data at a single-cell resolution that simultaneously measures cellular function and spatial coordinates. CCPLS performs estimation for all cell types of interest, allowing comprehensive investigation of the associations between cell types and gene expression all at once.
Market Application
CCPLS is applicable to spatial transcriptome data at a single-cell resolution in general and can extract genes that show expression changes depending on the spatial arrangement of cell types. For example, CCPLS is expected to be applied to drug target discovery focusing on the microenvironment of cells, such as cold tumors that are considered to have high malignancy and few surrounding immune cells. In recent years, some international consortiums have been collecting and accumulating large-scale spatial transcriptome data. Applying CCPLS to these data is expected to lead to the discovery of various biological findings, from basic biology to disease research.
Publications
https://academic.oup.com/bioinformatics/article/38/21/4868/6692423
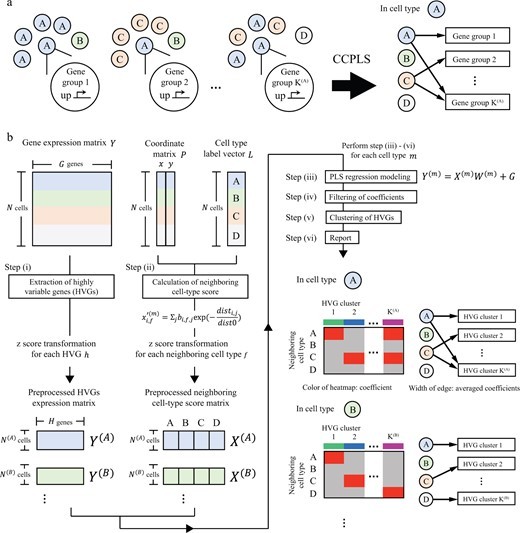
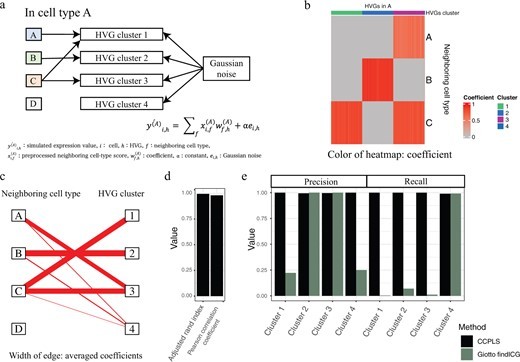
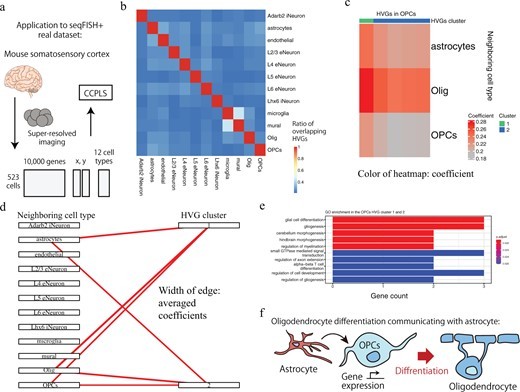
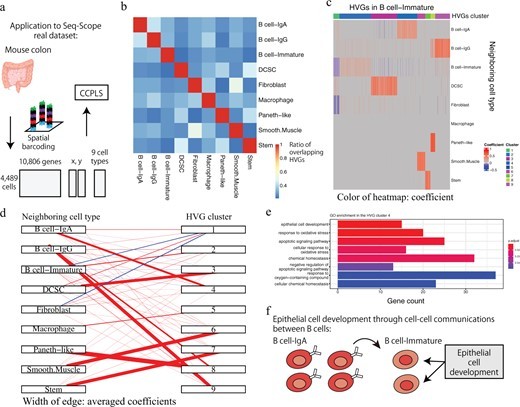